Written By:
Gopal Erinjippurath
Populous: Unpacking the geospatial dimension for multimodal insights
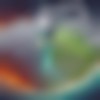
By Gopal Erinjippurath, Co-Founder & CTO at Sust Global and David Schottlander, Product Lead at Google Research
Executive summary
Legacy risk and economic models, grounded in historical relationships, consistently understate the pace and volatility of climate-driven change. These models aren’t capturing climate impacts on investor returns.
Geospatial AI using multimodal data provides a powerful set of tools to incorporate population dynamics, socioeconomic variables and climate risk factors enabling predictions on granular financial materiality and insurance market metrics.
Institutional investors can enhance decisions with increased visibility into future market trends and apply geospatial AI driven inference across risk management, portfolio optimization and scenario analysis workflows.
Blind spots across investment workflows
When billion-dollar portfolios hinge on yesterday’s models, even well-capitalized institutions can find themselves exposed. Institutional investors today face a rapidly changing risk landscape that traditional models struggle to capture. From unprecedented climate-related losses to complex global market dynamics, existing financial modeling frameworks often lack the granularity and foresight needed for truly informed decision-making. For example, U.S. home insurance premiums surged by 33% nationwide from 2020 to 2023, with some regions like Florida and California seeing over 50% spikes. Major insurers pulled out of high-risk areas, leaving homeowners—and by extension, mortgage lenders and property investors—exposed to cascading financial risks. These kinds of climate-driven shocks underscore the urgent need for more advanced, data-driven models that can anticipate and quantify emerging threats before they destabilize institutional portfolios.
Yet, current risk analysis workflows are riddled with blind spots. Key data on evolving perils (like insurance non-renewal rates or granular loss metrics) have historically been scarce or fragmented in the public domain. This opacity makes it difficult for analysts to fully assess how phenomena like climate change impact asset values and insurance availability.
Legacy risk and economic models, grounded in historical relationships, consistently understate the pace and volatility of climate-driven change. To navigate this uncertainty, investors must shift from backward-looking assumptions to forward-looking scenario frameworks that capture a range of plausible futures. In turn, institutional investors—pension funds, banks, insurers, hedge funds—find it challenging to incorporate these tail risks into their strategies. The result is often reactive decision-making and inefficient capital allocation, as firms rely on coarse averages or outdated assumptions.
The promise of Geospatial AI
Over the past 24 months, several geospatial Foundation Models (geoFMs) have emerged—such as Prithvi EO from IBM, Clay from Radiant Earth Foundation, and ClimaX/Aurora from Microsoft—promising to revolutionize geospatial AI by providing powerful, pretrained representations of the physical world. However, their applications in real-world scenarios are in their early days, and they have yet to fully enable frontier decision-makers in critical industries like finance, insurance, and infrastructure planning.
Google has been exploring how to create representations of the rich fabric of data related to population dynamics, such as search trends, map-based places information and environmental data like weather and air quality, in a way that is privacy preserving and amenable to machine learning in geospatial contexts. The result of this is a population dynamics foundation model and resultant embeddings (PDFM) that have recently been made available. You can read more details here.
In this context, we are excited to introduce a new geospatial AI-driven modeling framework for predicting socioeconomic and financial impact metrics. Populous – introduced in a recent research study – is the first US-wide AI framework capable of predicting critical insurance market metrics at fine spatial resolution. This framework can transform institutional investor workflows, enabling proactive risk management and smarter portfolio decisions. You can read the full technical paper here.
Key Findings
The research behind Populous demonstrates how innovative geospatial AI techniques can unlock deeper insights for financial risk modeling.
Below we summarize the core findings and technical breakthroughs from the paper, highlighting why this framework represents a leap forward:
Multimodal Data Integration: The framework ingests a wide array of data — from demographic and economic indicators to detailed climate risk metrics and even signals from a Google-developed foundation model. By combining diverse data sources, the model builds a rich, context-aware representation of risk that far surpasses siloed traditional approaches. Notably, it leverages PDFM embeddings capturing human and environmental patterns to inject learned knowledge about local population dynamics directly into the predictions. This transfer learning approach means the model can pick up subtle geospatial relationships and human behavioral trends that conventional financial models would miss.
Figure 1: The Populous model is composed of a multi-stage architecture used for predicting home insurance premiums and non-renewal rates. The first stage (level 1) trains a county-level predictive model using multimodal inputs, followed by a second stage (level 2) applying zipcode-level super-resolution to refine predictions with county-level outputs and additional fine-grained features.
Two-Stage “Coarse-to-Fine” Modeling: Populous employs a novel two-tier architecture to achieve high-resolution predictions. In Stage 1, a model is trained on county-level data to capture broad spatial trends in insurance outcomes. In Stage 2, those predictions are used as inputs (along with additional fine-grained features) to produce zipcode-level outputs, essentially performing an embedding-based super-resolution of the results. This design allows the framework to learn from wider patterns where data is plentiful, then drill down to local nuances. The result is unprecedented spatial precision in the risk estimates. Even in counties with sparse or no historical data, the model can infer “plausible” premium and non-renewal rates by learning from analogues elsewhere. Such granular insight was previously unattainable at a national scale. By capturing localized variations in risk and pricing, the framework bridges the gap between macro-level models and on-the-ground reality, making it highly applicable to real-world decision-making.
Superior Predictive Performance: The new framework isn’t just innovative in theory – it delivers quantifiable improvements in predictive power. In tests, the model achieved 93% top-2 accuracy in classifying county-level insurance premium categories, effectively reproducing the spatial distribution of premiums across the United States. It also explained over 61% of the variance in county-level policy non-renewal rates, a key indicator of market stress. These figures represent a significant improvement over currently available benchmarks. Moreover, even when applied to unseen regions, the model’s predictions aligned closely with real-world outcomes, giving confidence in its generalizability. At the zipcode level, performance tacks closely with records – about 89.5% top-2 accuracy for premiums and ~50% variance explained for non-renewals in test cases – demonstrating that fine-scale prediction is feasible with this approach. This level of accuracy and resolution can materially improve risk forecasting, enabling analysts to trust the model’s outputs for high-stakes decisions.
Figure 2: Model performance in reproducing observed 2023 insurance premiums (top) and non-renewals (bottom) across the U.S. at the county level.
Insight into Key Risk Drivers: Beyond raw performance, Populous offers transparency into the drivers of risk – a critical feature for institutional adoption. Feature importance analyses reveal that the model heavily weights climate-related variables when predicting insurance trends. In fact, historical climate events such as past wildfire and hurricane occurrences and projected future climate risks rank among the top factors influencing premium increases and non-renewals. This finding aligns with domain intuition that areas facing higher physical hazards are seeing the greatest insurance turmoil. It also quantifies that intuition: when we removed climate hazard features, the model’s performance dropped dramatically, a 17% decline in explanatory power at the county level. Together, these results underscore the need to analyze climate risk alongside socioeconomic factors to evaluate drivers of financial outcomes in the housing and insurance market. For investors, this offers a data-backed and explainable view into how and where climate factors are hitting portfolios hardest.
Figure 3: Past climate hazards and future climate risk are among the most influential features for nationwide county-level premiums (left) and non-renewals (right), particularly tropical cyclones (hurricanes).
Flexible and Extensible Framework: Another strength of the framework is its adaptable design. While the initial study focused on home insurance metrics, the underlying architecture can be readily extended to other targets and markets. The model is built to handle various types of output variables – whether predicting insurance losses, housing vacancy rates, real estate values, loan default rates, or even demographic shifts. Because it’s fundamentally a general multimodal learner with a super-resolution capability through fine-tuning PDFM, one could retrain or fine-tune this model to predict different impact variables relevant to institutional portfolios. This flexibility means that Populous is not a one-off model, but a new framework for financial AI – one that can evolve with emerging data and be tailored to specific use cases in investment management.
Feature importance analysis confirms that climate-related factors (e.g. hurricane exposure, wildfire risk) are among the top drivers of insurance outcomes. In the nationwide model, tropical cyclone metrics and past wildfire events rank at the very top for both premium increases and policy non-renewals. This interpretability gives risk analysts confidence in the model’s outputs and helps pinpoint why certain regions are more vulnerable. Such insights enable proactive strategies – for instance, an investor can recognize that an area’s surging non-renewal rate is fundamentally tied to escalating wildfire risk, and act accordingly.
Applications in Institutional Workflows
The Populous framework provides a practical tool poised to augment institutional investor workflows. By integrating this modeling framework into their active risk and opportunity sizing processes they can dramatically enhance how they assess risk, forecast market changes, and optimize portfolios.
Key applications areas include:
Risk modeling and portfolio screening: Institutional risk managers can use the model’s granular predictions to identify emerging risks at an early stage. By quantifying risks that were previously qualitative or hidden, organizations can proactively adjust underwriting criteria, reserve buffers, or insurance coverage in high-risk locales well before crises hit. By integrating these risk factors into financial models (e.g. DCF valuations or capital allocation plans), firms can price in future risk more accurately when modeling capitalization rates, yields and exit valuation. The framework enables a more forward-looking risk analysis, turning complex climate and socioeconomic data into concrete financial outlooks. This empowers investment committees to make informed strategic choices under uncertainty, backed by AI-driven evidence.
Portfolio optimization: Perhaps the most consequential use of this framework is in optimizing investment portfolios for risk-adjusted returns. Institutional investors often juggle large portfolios of real estate assets, mortgages, municipal bonds, and equities—many of which have geographically linked risk (think housing loans in wildfire zones or utility stocks serving hurricane-prone regions). With Populous, portfolio managers can quantify the previously opaque risks in each asset and region. For instance, a pension fund could overlay the model’s zipcode-level risk scores on their real estate holdings to identify which properties might become uninsurable or severely expensive to insure in the near future. This could inform decisions to rebalance holdings, divest from high-risk area assets, or increase allocations to regions projected to remain resilient.
Scenario analysis: Because Populous captures the relationship between environmental factors and financial outcomes, it is ideal for scenario planning. Analysts can pose “what if” questions – such as, How would a 20% increase in wildfire incidence or a major hurricane landfall affect our portfolio’s risk profile? – and let the model simulate impacts on insurance losses, premiums, or loan non-payment rates. It also aids forecasting of market trends: an asset manager could project how insurance costs might rise over the next 5–10 years in various regions, and how that might influence home values or consumer spending.
From research to cloud-native solutions
The introduction of the Populous multimodal AI framework is an exciting next step on how we as a community tackle financial risk, opportunity and portfolio management. Embracing such advanced modeling tools can provide a competitive advantage in an era of increasingly complex multimodal risk factors.
Populous will be presented at ICLR2025 in Singapore. You can read the full technical paper here. Sust Global’s work in geospatial AI is being featured in sessions from Google Research at Google Cloud Next25 in Las Vegas as part of their AI Research in Action showcase.
Populous will be integrated with Sust Global’s geospatial AI solutions in Q2 2025.
We invite institutional investors and technical AI professionals to engage further on this research. Whether you’re a risk officer looking to enhance your models or an AI specialist interested in real-world financial applications, now is the time to explore how this framework can be put into practice. We encourage industry professionals to dive into the details of the methodology, examine the open research and results, and consider piloting these techniques within your organizations. Don’t wait for the next crisis to expose the weaknesses in outdated models. Reach out and get in touch to learn more about implementing Populous and related AI solutions in your workflow.
By collaborating and experimenting with these new tools, you can help usher in a new era of data-driven, resilient, and optimized financial decision-making. The opportunity to stay ahead of the curve is here – seize it, and lead your institution into the future of intelligent investing.