A response to Bloomberg: All climate models are wrong, but some are useful
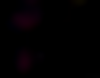
Commercial climate risk companies like Sust Global provide data and analytics on present day and future climate risk exposure. This data helps reporting and analytical teams screen, quantify and report on the financial impacts of acute and chronic physical climate hazards. A recent article from Bloomberg has highlighted concerns around disagreements and transparency of climate models.
The article lays out three key concerns regarding climate risk companies and their data: Firstly, comparing across risk projections is challenging. Secondly, there are disagreements across models like those from the research team at UC Irvine and climate risk companies when comparing high resolution projections. Lastly, precise predictions at the property level are not reliable.
The recent article from Bloomberg rightly points out variability and a lack of consensus across commercial risk projection models. However, there are important nuances that have been overlooked while exploring such comparisons:
Uncertainty measures enable fair comparison
The Bloomberg article outlines the challenges with comparing across model projections. However, it fails to portray climate risk models (e.g. flood, cyclone or wildfire models) for what they really are – probabilistic models with uncertainty.
Climate risk models estimate the likelihood and severity of hazardous impact from climate extremes, with most commercial climate risk models deriving their results from the CMIP6 (Coupled Model Intercomparison Project Phase 6) models. CMIP6 is an international climate modeling initiative that coordinates and compares fundamental climate attributes from various global climate models to improve understanding of climate change. By design, the CMIP6 models tend to see greater consensus across the scientific community as described in the IPCC reports (link).
On the other hand, there is no standardized way in which climate risk models are required to weight fundamental climate attributes such as temperature, precipitation and surface pressure. This results in greater variability across hazard projections from climate risk models. Commercial climate risk data providers like Sust Global address this variability through using a mixture of physics-based modeling and statistical/AI-based modeling. To enable fair comparison across climate risk models, climate modeling firms must provide uncertainty measures on climate risk projections, enabling better comparisons across modeled outcomes.
Capturing variability across space
The article correctly outlines climate risk as spatially varying and how different vendors account for spatial variability differently. Remote sensing approaches allow us to account for spatial variability for hazard projections.
There is abundant literature on different approaches to using remote sensing to account for an understanding of the Earth system such as terrain elevation for flood models and land cover types and vegetation for wildfire models. However, this also leads to modeling teams accounting for spatial variability in different ways leading to variations in their projections as highlighted in the article.
Improved validation for hyper local climate risk measures
There is no “perfect” way to model climate risk yet. It is an area of active research and product development. Assumptions and initial conditions govern the projection results.
The cited paper highlights the urgent need to systematically monitor urban flooding at hyper-local scales for better validation. A class of validation methods is against a historic catalog of climate hazard events, mapping asset level exposure and impact from satellite derived observations of acute hazards such as wildfire, floods and tropical cyclones.
Conclusion
Climate models are complex. While commercial solutions will continue to be proprietary with the exact methodologies being protected IP through either patents or trade secrets, there needs to be standardization on predictive metrics and their meaning used to communicate climate risks from physical hazards.
Decision makers and capital allocators accounting for climate risks “need” risk projections at the property level to make them useful to their decision making process and analytical workflows. When they make the informed trade off between consensus driven model projections with spatial imprecision or quantified uncertainty at high spatial precision, our experience has been that they tend to the latter.
At Sust Global, we have provided our climate risk data to multiple academic researchers in the past, resulting in peer-reviewed research available to all. The recent publication from the researchers at Monash University in the Journal of Ecological Economics is one such example. We've also published our AI-based methodologies and benchmarking results built on top of the underlying proprietary data to enable greater visibility into our benchmarking and validation approaches, see our paper from ICLR2023.
To learn more about our climate risk datasets and analytics and our validation methodologies, reach out to us at contact@sustglobal.com or submit a query below.
References
Clashing risk predictions cast doubts on black box climate models (Bloomberg Green, link)
The risky business of predicting where climate disaster will hit (Bloomberg Green Big Take, link)
National-Scale Flood Hazard Data Unfit for Urban Risk Management, AGU, Earth’s Future, July 2024 (link)
Climate Change and its impact on home insurance uptake in Australia, Aug 2024 (link)